Data 102: Data, Inference, and Decisions
UC Berkeley, Fall 2024
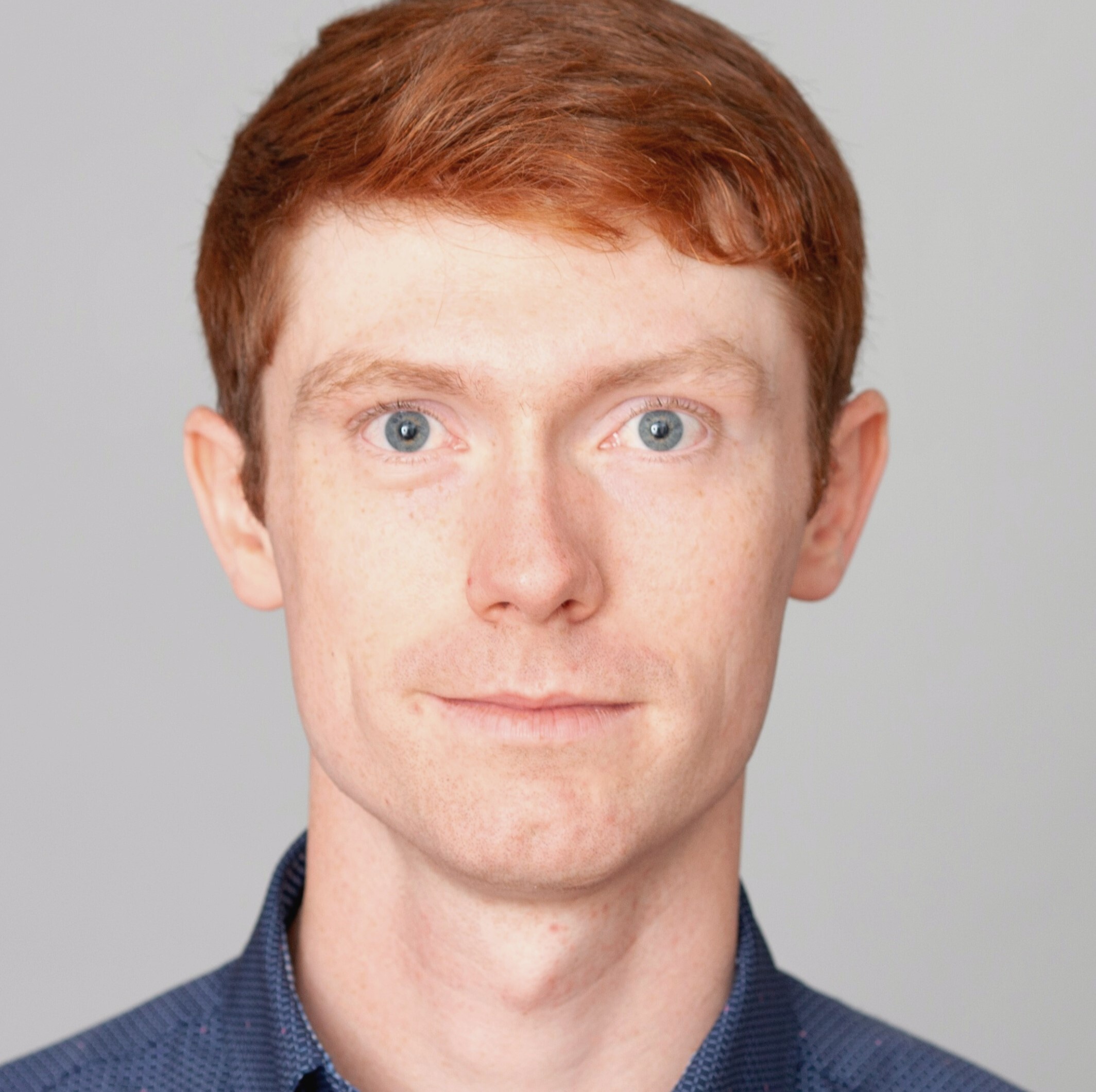
Alexander StrangInstructor
alexstrang
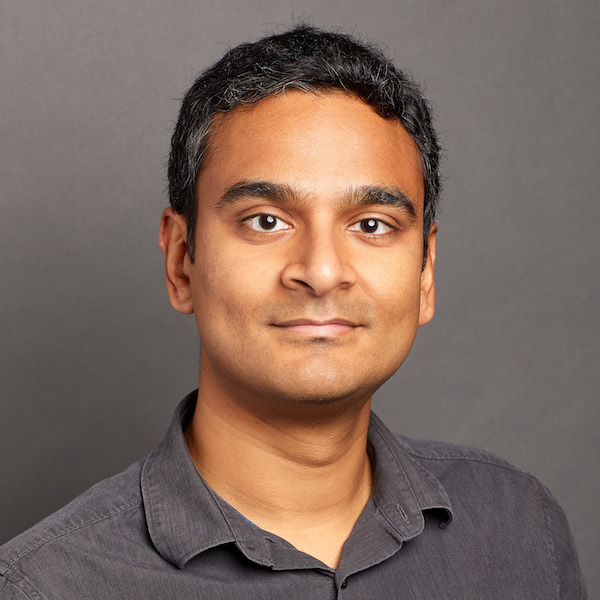
Ramesh SridharanInstructor
ramesh_s
Schedule
- Aug 29
- Lecture 1. Binary Decision-Making I
- Vitamin Vitamin 1 (due Sep 4 at 11:59 PM)
- Sep 2
- Lab Lab 1: Review and Warm-Up (due Sep 4 at 5 PM)
- Sep 3
- Lecture 2. Binary Decision-Making II
- Discussion Discussion 1 (Answers)
- Sep 5
- Lecture 3. Binary Decision-Making III: Hypothesis Testing
- Vitamin Vitamin 2 (due Sep 8 at 11:59 PM)
- Sept 6
- Homework Homework 1 (due Sept 20 at 5 PM)
- Sep 9
- Lab Lab 2: Testing (due Sep 11 at 5 PM)
- Sep 10
- Lecture 4. Specificity and Sensitivity (Benjamini Hochberg and Neyman Pearson)
- Discussion Discussion 2 (Answers)
- Sep 12
- Lecture 5. Decision Theory (Loss and Risk, Frequentist and Bayesian)
- Vitamin Vitamin 3 (due Sep 15 at 11:59 PM)
- Sep 16
- Lab Lab 3: Loss and Risk (due Sep 18 at 5 PM)
- Sep 17
- Lecture 6. Parameter Estimation and Inference: Introduction to Frequentist and Bayesian Modeling
- Discussion Discussion 3 (Answers)
- Sep 19
- Lecture 7. Bayesian Hierarchical Models
- Vitamin Vitamin 4 (due Sep 22 at 11:59 PM)
- Sept 20
- Homework Homework 2 (due Oct 4 at 5 PM)
- Sep 23
- Lab Lab 4: Graphical Models (due Sep 25 at 5 PM)
- Sep 24
- Lecture 8. Bayesian Hierarchical Models II
- Discussion Discussion 4 (Answers)
- Sep 26
- Lecture 9. Bayesian Inference with Sampling
- Vitamin Vitamin 5 (due Sep 29 at 11:59 PM)
- Sep 30
- Lab Lab 5: Sampling & GLMs (due Oct 2 at 5 PM)
- Oct 1
- Lecture 10. Sampling and Prediction
- Discussion Discussion 5 (Answers)
- Oct 3
- Lecture 11. GLMs
- Vitamin Vitamin 6 (due Oct 6 at 11:59 PM)
- Oct 7
- Lab Lab 5.5: GLMs (due Oct 11 at 5 PM)
- Oct 7
- Review Session and MiniLab Slides
- Oct 8
- Midterm Midterm 1
- Oct 10
- Lecture 12. Uncertainty Quantification for GLMs
- Oct 11
- Homework Homework 3 (due Oct 25 at 5 PM)
- Oct 14
- Lab Lab 6: GLMs and the Bootstrap (due Oct 16 at 5 PM)
- Oct 15
- Lecture 13. Nonparametric Methods and Neural Networks
- Discussion Discussion 6 (Answers)
- Oct 17
- Lecture 14. Neural Networks and Interpretability
- Vitamin Vitamin 7 (due Oct 20 at 11:59 PM)
- Oct 21
- Lab Lab 7: Nonparametric methods (due Oct 23 at 5 PM)
- Oct 22
- Lecture 15. Causal Inference I: Association and Causation
- Discussion Discussion 7 (Answers)
- Oct 24
- Lecture 16. Causal Inference II: Randomized Experiments
- Vitamin Vitamin 8 (due Oct 27 at 11:59 PM)
- Oct 25
- Homework Homework 4 (due Nov 8 at 5 PM)
- Oct 28
- Lab Lab 8: Instrumental variable (due Oct 30th at 5 PM)
- Oct 29
- Lecture 17. Causal Inference III: Observational Studies
- Discussion Discussion 8 (Answers)
- Oct 31
- Lecture 18. Concentration Inequalities and Tail Bounds
- Vitamin Vitamin 9 (due Nov 3 at 11:59 PM)
- Nov 4
- Lab Lab 9: Unconfoundedness (due Nov 6 at 5 PM)
- Nov 5
- Lecture 19. Bandits I
- Discussion Discussion 9 (Answers)
- Nov 7
- Lecture 20. Bandits II
- Vitamin Vitamin 10 (due Nov 10 at 11:59 PM)
- Nov 8
- Homework Homework 5 (due Nov 22 at 5 PM)
- Nov 12
- Lecture 21. Reinforcement Learning I
- Nov 13
- Review Session Slides
- Nov 14
- Midterm Midterm 2
- Vitamin Vitamin 11 (due Nov 17 at 11:59 PM)
- Nov 17
- Lab Lab 10: Bandits (due Nov 20 at 5 PM)
- Nov 19
- Lecture 22. Reinforcement Learning II
- Discussion Discussion 10 (Answers)
- Nov 21
- Lecture 23. Monte Carlo Tree Search
- Nov 25
- Homework Homework 6 (due: first half Dec 3 at 3:30 PM, full assignment Dec 6 at 5 PM)
- Nov 26
- Lab Lab 11: Reinforcement Learning (due Dec 4 at 5 PM)
Lab Lab 12: Differential Privacy (Optional) (No due date)
- Nov 26
- Lecture 24. Case Studies, Robustness, and Generalization
- Nov 28
- Lecture Holiday
- Dec 3
- Lecture 25. Bridging Technical & Ethical Perspectives on Modeling and Decisions
- Dec 5
- Lecture 26. Course Wrap-Up